This article is part of a series – the contents page is here.
Let’s start somewhere near the beginning with a few definitions:
- A position is the state of the chess board at a given point. It includes the locations of the pieces and the knowledge of whose turn it is to move next. It also includes subtle stuff like whether the players have moved their kings or rooks, which affects their ability to castle.
- A move is a single move by one player, such as White pushing the pawn from e2 to e4. Each move takes a position and creates a new position.
- A line is a sequence of moves. For example, the line 1. e4 e5 2. Nf3 Nc6 3. Bb5 is the start of the Ruy Lopez opening.
We’ll assume that we have a function that understands the rules of chess and can tell us the legal moves in any given position. That’s not too hard.
We’ll also assume that we have a function that can look at a position and give it a score, such that a more favourable position will be given a higher score than an undesirable position. That’s not easy at all! But we’ll assume that we have it anyway, and we’ll call it our “evaluation function.”
We need to be quite precise with our definition of a “higher” score. First we need to recognise that what’s good for White is bad for Black and vice versa. So if a position is good from White’s point of view (and thus should have a “higher” score) then it is bad from Black’s point of view (and thus should have a “lower” score).
We adopt the convention that a highly positive score is good for White and a highly negative score is good for Black. We also adopt the benchmark that a pawn is worth 100 points. So if the evaluation function gives a position a score of +100 then that means that White has an advantage equivalent to being up by one pawn. (The advantage need not be material, White’s pieces might simply be better placed, giving a moderate positional advantage). A position score of -100 would mean that it was Black who had the advantage.
The evaluation function
The evaluation function is the “secret sauce” of a chess engine. A serious evaluation function will account for all kinds of subtle positional factors and give them carefully considered weightings. We’re not going to do anything as sophisticated as that here. The good news is that you can make a non-trivial chess engine with just a few simple heuristics. The most obvious, and easiest, of these is a naïve tally of material. Using the time-honoured scores of pawn=100, knight=330, bishop=350, rook=500 and queen=900, we simply add up the material in the position (using positive numbers for White and negative numbers for Black). If both sides have the same pieces then this will give us a zero score.
The other simple thing we can do is to make the function aware of checkmate. A position in which the opponent has been mated trumps any other position so it deserves a score of infinity (negative infinity in the case of Black). We’ll add a bit more intelligence to our evaluation function later.
The minimax algorithm
Imagine that we are a computer chess player and it’s our turn. We want to play the best possible move. Armed with the current position, our move generator and our evaluation function, we can do what computers do best: search. The obvious way to find our best move goes like this:
- For all of our legal moves:
- Apply the move to the current position to obtain a new position.
- Run that new position through the evaluation function and get its score.
- If that score is better than the best score we’ve seen so far then the move becomes our new best move and its score becomes our new best score.
- Play the best move and we’re done.
If it were that simple then chess wouldn’t be a very interesting game. What makes it complex, of course, is that we have to consider our opponent’s response to our move. Consider the standard position below, where it’s White’s turn to move. Following the algorithm above, and using our simple materialistic evaluation function, we would conclude that the “best” move is for the knight on f3 to take the black pawn on e5, since that’s the only move that produces a position where White has a material advantage.
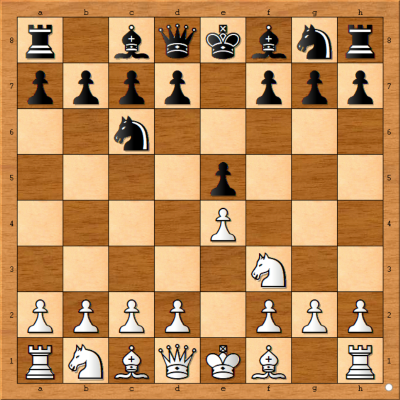
But of course Black can respond by taking the white knight with the knight on c6. What looked like the best move when we searched the move tree to a depth of one became the worst move when we searched the tree to a depth of two! This is known as the horizon effect and it was an important reason why early engines (running on the relatively slow hardware of the ’70s and ’80s) were quite beatable by Grandmasters.
So how can we extend our algorithm to take our opponent’s replies into account? The first thing to notice is that our opponent has many potential replies to each of our moves. In the above example, we saw that Black could reply to 1. Nxe5 with 1. .. Nxe5. But Black could also have responded with 1 .. d6, or any of a bunch of possibilities. To get anywhere, we have to assume that our opponent will play the move that gives the maximum advantage for him/her (or, from our point of view, the worst score for us). To put it another way:
- Our best move is the one that minimizes our opponent’s maximum advantage.
This idea is what gives the famous algorithm its name: minimax. And I hope you can see that the same process will still work when we go deeper and consider our replies to our opponent’s replies to our replies to our opponent’s replies… In theory, the deeper we go in our search, the better our eventual move will be. We might find a move that looks good at first, but when we consider all the replies to a depth of, say, five moves, we might discover a refuting reply that changes our assessment of the original move.
Notice also that we don’t need to run the evaluation function on every position (or ‘node’, to use the search tree parlance). We evaluate only the ‘leaf’ nodes – the positions where we decide not to search any deeper (plus the positions that represent the end states of checkmate and stalemate). The score that we get from evaluating a leaf node represents the score for the entire line of moves that define the branch of the tree we have searched. We’re going to play the move that starts the line that gives us the best score.
The alpha-beta algorithm
The minimax algorithm will find the best move of the subtree that we search (where “best” is defined by the evaluation function), but it has one enormous problem. On a move tree as vast as the game of chess, it’s just way too slow. We need to find ways to prune that tree. Fortunately there’s an easy one available that will give us a significant improvement in many situations.
Consider the position below. We’re White and it’s our turn to move. We need to find the best move, and fast!

Notice that the minimax algorithm told us to consider all of our moves, but it didn’t say anything about the order in which we should look at them. Now that we’re trying to improve performance, intuition suggests that it might help if we start by considering the ‘heavy hitter’ moves – such as captures and checks. This would mean that we would soon look at the two checking moves: 1. Nd6 and 1. Nc7. And it wouldn’t take long to see that 1. Nc7 is a terrific move! The best Black can do is 1. .. Kf8, followed by 2. Nxd5, winning the queen. Even our naïve evaluation function will give this position a score of about +900 because Black has lost that 900 point queen.
And here is where we can start to short-circuit the minimax algorithm’s slow search. Suppose that we next consider a boring move for White like 1. a3. As we look through Black’s replies we will find a move like 1. .. Qc5. Now that is not a great move, but you know what? It doesn’t lose the queen, so its evaluation is less than +900. In other words, we have quickly discovered that if we play 1. a3, Black has a reply that will leave him/her better off than he/she would have been if we had played 1. Nc7. That’s all we need to know: we can quickly reject the whole move. Sure, if we kept searching we would eventually discover that 1. .. Qd8 is an even better reply for Black than 1. .. Qc5, but who cares?! We already know that 1. a3 is not as good for White as 1. Nc7.
This is known as the alpha-beta algorithm. It produces the same answer as the minimax algorithm, but is often faster. The name comes from the two scores that allow us to short-circuit the search. Alpha is that terrific +900 score that we found when we looked at 1. Nc7. Beta is the score for the line that starts with 1. a3 Qc5. Because it’s worse (from White’s point of view) than +900 it enables us to discard 1. a3 quickly.
Notice that the alpha-beta shortcut works best when we find a great score early in our search. The higher the score and the sooner we find it, the better. That’s why the order in which we consider the moves is important.
Here’s the bit where your head might start to spin: alpha-beta works at any depth. Even though we started out searching for White’s best move, we can still use the alpha-beta shortcut when considering Black’s replies. At every depth n we keep track of the best score we’ve seen so far and for each move we consider we pass that alpha score to the reply search at depth n+1. That’s exactly what local variables in recursive functions are for!
The important thing to remember at each level is to evaluate the score from the player’s point of view. When we’re trying to find Black’s best reply to White’s first move our alpha will be the most negative score we’ve found. At the next level (which evaluates White’s replies to Black’s reply to White’s first move), we’ll bail out if we find a beta score that is greater than that alpha score (since that would mean that White has a reply that leaves White better off and Black worse off than Black would be if he/she played his/her alpha move instead).